About
This success story originates from a Master’s thesis at the University of Aveiro, which explored algorithms for river discharge forecasting. Accurate river discharge forecasting plays a crucial role in water quality control, flood prevention, risk evaluation, the design of hydroelectric dams, and the management of reservoirs and water scarcity. Moreover, recent years have seen significant shifts in climatic patterns due to climate change, leading to an increase in extreme weather events. This shift underscores the need for improved forecasting capabilities to support decision-making processes and to mitigate the consequences of these events.
However, hydrologic data, such as river discharge measurements, is not always readily accessible, and obtaining it independently can be prohibitively expensive, making it unavailable to many institutions. This research aims to develop and publicly provide a model capable of reliably and accurately representing temporal variations in river discharge. Additionally, with a focus on accessibility, this study seeks to determine whether it is possible to approximate the quality of forecasts produced by models that have access to hydrologic data by using only widely available data, such as temperature and precipitation records.
The Challenge
The main challenge of this research was optimizing hyperparameters. Identifying the ideal combination required training the models for each possible set of hyperparameters and then analyzing the outcomes. Given that this research explored three AI models across four rivers, three instances, and four different timespans, the total training time reached thousands of hours. The computational resources required far exceeded those available on standard personal computers.
The Solution
The solution involved leveraging high-performance computing (HPC), specifically using multiple GPU cores to parallelize the training process. This approach significantly reduced the training time and enabled a broader exploration of hyperparameter combinations during the optimization phase.
Services Provided
The pilot project HPCvLAB@CVA-UA bridged the computational needs of this Master’s thesis with HPC resources provided by EUROCC/INCD. This pilot project offers 300.000 core hours across multiple operational centers, of which 233.75 core hours were utilized during the optimization phase.
Impact
This access to HPC resources made it possible to run essential experiments, which played a pivotal role in the successful completion of this Master’s thesis.
Involved Partners
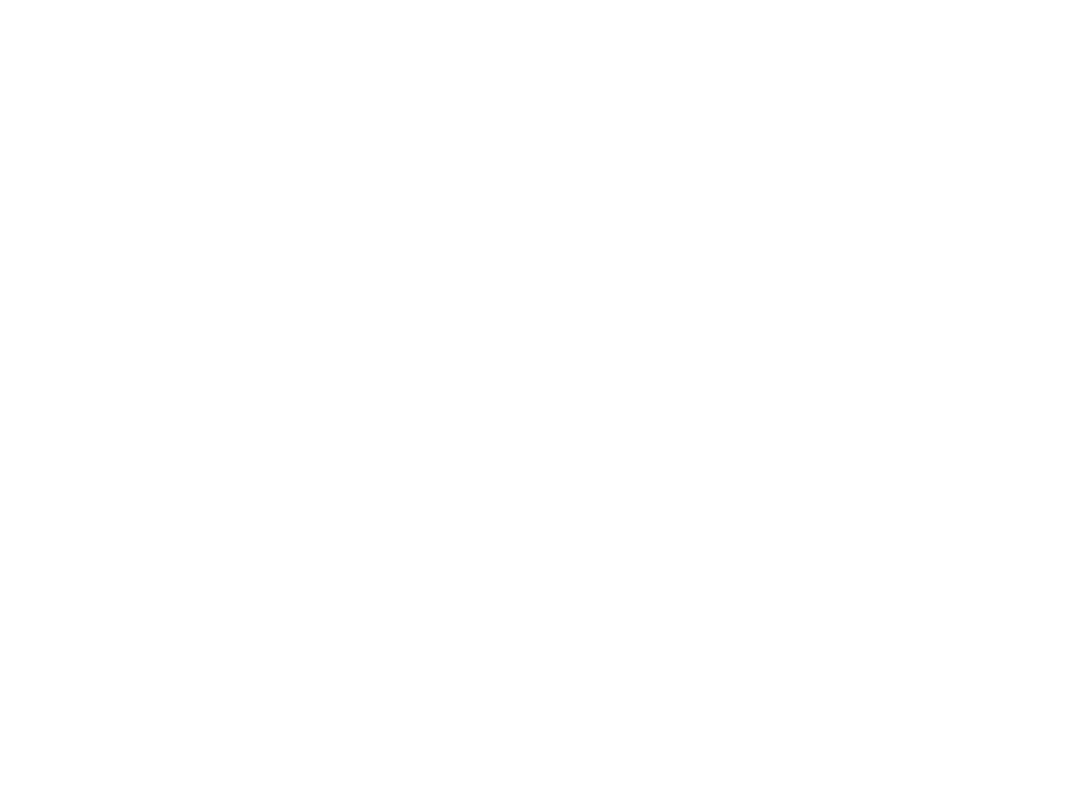
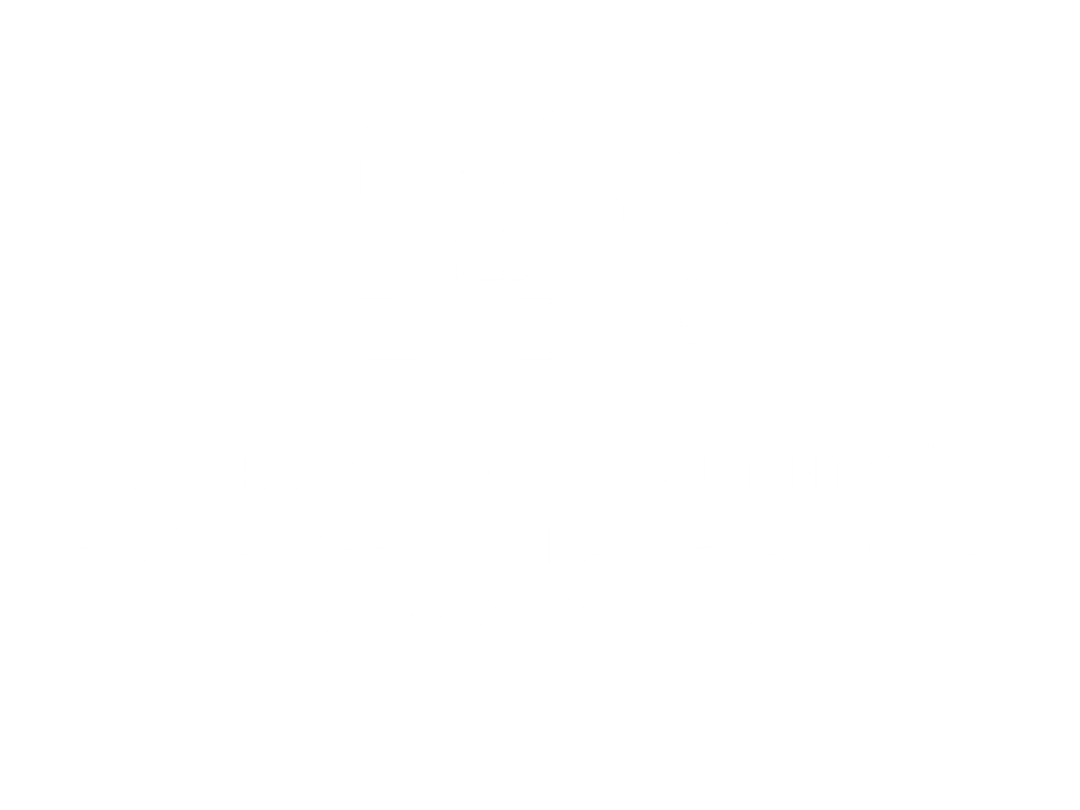
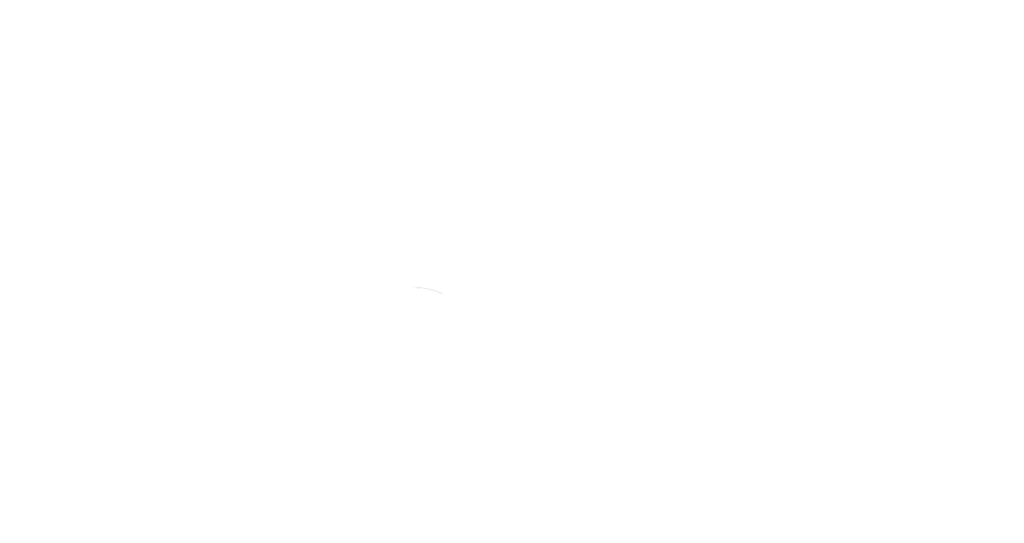