@MoreiraLAB www.moreiralab.com
Our project, VIRUSHOSTAI – DSAIPA/DS/0118/2020, is a good example of the advantage of using High Performance Computing as it involves dynamic simulations of proteins as well the most recent algorithms of deep-learning. We are following a network-based approach to predict new links between virus and their hosts, essential towards the establishment of a new molecular data-driven precision medicine. This project was awarded within the framework: “AI 4 COVID-19: Data Science and Artificial Intelligence in the Public Administration to strengthen the fight against COVID-19 and future pandemics – 2020”.
Unfortunately, we are all familiar with Severe Acute Respiratory Syndrome CoronaVirus-2 (SARS-CoV-2), a member of the Coronaviridae family, responsible for the COronaVIrus Disease (COVID-19), a pandemic ravaging the world for the last two years. Since its first report in December 2019 in Wuhan, China, over 355 M infection cases and more than 5.6 M deaths were reported worldwide (as of January 25th, 2022).
One of the most conserved structural proteins in SARS-CoV-2 is the Membrane (M) protein, as it has a smaller mutation rate and shares structural and functional similarities with M proteins from other coronaviruses. This protein has several functions and, despite some not being yet fully understood, it plays an important role on the viral infection. In fact, M protein is a central player in the virion formation through interactions with itself (upon the formation of a homodimer) and to other structural proteins and lipids. M protein’s structure has not yet been determined experimentally, but AlphaFold released its prediction early on the pandemic, which served as the basis of our work. We developed a detailed in silico protocol to predict its homodimeric structure and correct membrane orientation and to analyze structural and dynamic effects of reported M protein mutations. Furthermore, we predicted the mutation impact on potential druggable residues, specifically on the dimer interface area.
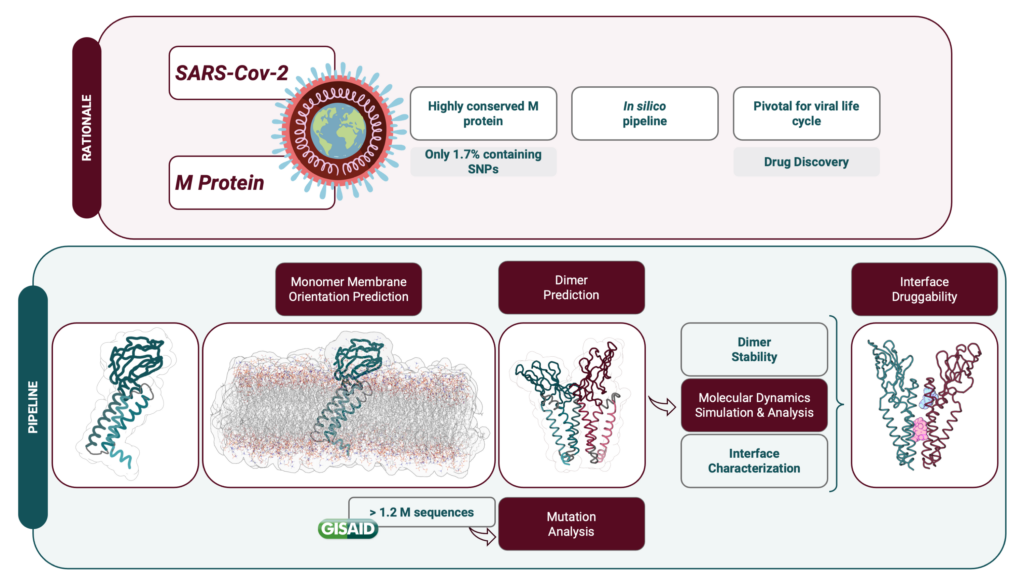
In this study we clearly extended the knowledge on the structure-function relationship of the M protein and predicted new druggable pockets, revealing the therapeutical potential of this biological target. We are now using computational expensive artificial intelligence algorithms to build a data-driven approach that considers infectious diseases in the framework of complex networks emerging from the integration of information from various omics levels to identify new possible antiviral drug-proteins interactions. Due to the amount of computer power necessary, the use of the Cluster Navigator – Laboratório de Computação Avançada (LCA) from Coimbra University, will be key to pursue our aims.